Incomplete JSON Pretty Printer |
https://tools.simonwillison.net/incomplete-json-printer |
Every now and then a log file or a tool I'm using will spit out a bunch of JSON that terminates unexpectedly, meaning I can't copy it into a text editor and pretty-print it to see what's going on.
The other day I got frustrated with this and had the then-new GPT-4.5 build me a pretty-printer that didn't mind incomplete JSON, using an OpenAI Canvas. Here's [the chat](https://chatgpt.com/share/67dd9d55-7f70-8006-b55d-72730f60ddbe) and here's [the resulting interactive](https://chatgpt.com/canvas/shared/67e5e9b3f7bc8191b2306a123c9d328f).
I spotted a bug with the way it indented code today so I pasted it into Claude 3.7 Sonnet Thinking mode and had it make a bunch of improvements - [full transcript here](https://claude.ai/share/22dc4b58-e8c4-44a4-9650-a37d21513b8d). Here's the [finished code](https://github.com/simonw/tools/blob/main/incomplete-json-printer.html).
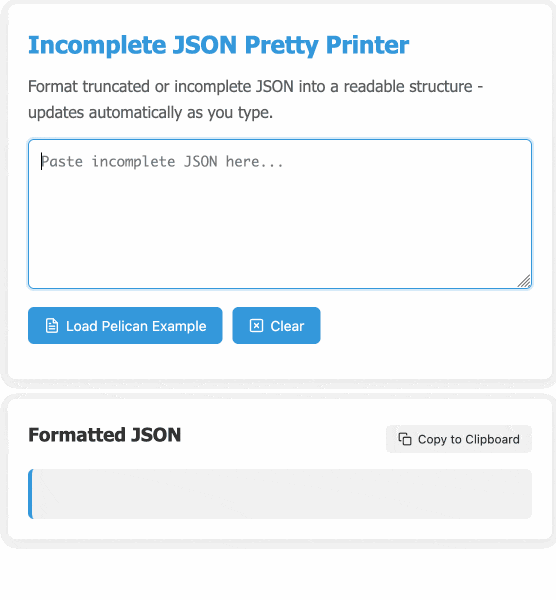
In many ways this is a perfect example of [vibe coding ](https://simonwillison.net/2025/Mar/19/vibe-coding/) in action. At no point did I look at a *single line* of code that either of the LLMs had written for me. I honestly don't care how this thing works: it could not be lower stakes for me, the worst a bug could do is show me poorly formatted incomplete JSON.
I was vaguely aware that some kind of state machine style parser would be needed, because you can't parse incomplete JSON with a regular JSON parser. Building simple parsers is the kind of thing LLMs are surprisingly good at, and also the kind of thing I don't want to take on for a trivial project.
At one point I told Claude "Try using your code execution tool to check your logic", because I happen to know Claude can write and then execute JavaScript independently of using it for artifacts. That helped it out a bunch.
I later dropped in the following:
> `modify the tool to work better on mobile screens and generally look a bit nicer - and remove the pretty print JSON button, it should update any time the input text is changed. Also add a "copy to clipboard" button next to the results. And add a button that says "example" which adds a longer incomplete example to demonstrate the tool, make that example pelican themed.`
It's fun being able to say "generally look a bit nicer" and get a perfectly acceptable result! |
2025-03-28 00:18:43+00:00 |
Tracing the thoughts of a large language model |
https://www.anthropic.com/research/tracing-thoughts-language-model |
In a follow-up to the research that brought us the [delightful Golden Gate Claude](https://simonwillison.net/2024/May/24/golden-gate-claude/) last year, Anthropic have published two new papers about LLM interpretability:
- [Circuit Tracing: Revealing Computational Graphs in Language Models ](https://transformer-circuits.pub/2025/attribution-graphs/methods.html) extends last year's interpretable features into [attribution graphs](https://transformer-circuits.pub/2025/attribution-graphs/methods.html#graphs), which can "trace the chain of intermediate steps that a model uses to transform a specific input prompt into an output response".
- [On the Biology of a Large Language Model](https://transformer-circuits.pub/2025/attribution-graphs/biology.html) uses that methodology to investigate Claude 3.5 Haiku in a bunch of different ways. [Multilingual Circuits](https://transformer-circuits.pub/2025/attribution-graphs/biology.html#dives-multilingual) for example shows that the same prompt in three different languages uses similar circuits for each one, hinting at an intriguing level of generalization.
To my own personal delight, neither of these papers are published as PDFs. They're both presented as glorious mobile friendly HTML pages with linkable sections and even some inline interactive diagrams. More of this please!
[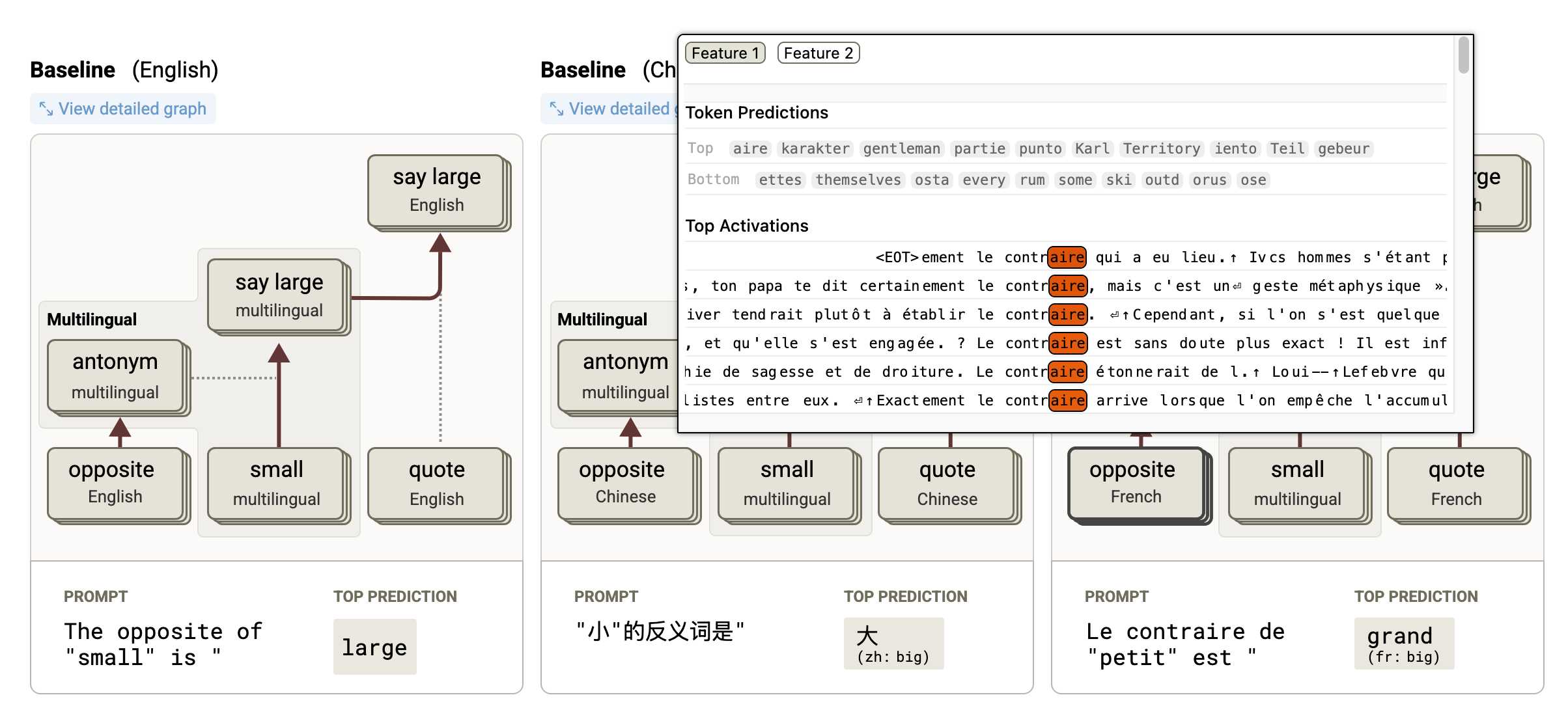](https://transformer-circuits.pub/2025/attribution-graphs/biology.html#dives-multilingual) |
2025-03-27 21:51:24+00:00 |
GPT-4o got another update in ChatGPT |
https://twitter.com/OpenAI/status/1905331956856050135 |
This is a somewhat frustrating way to announce a new model. @OpenAI on Twitter just now:
> GPT-4o got an another update in ChatGPT!
>
> What's different?
>
> - Better at following detailed instructions, especially prompts containing multiple requests
> - Improved capability to tackle complex technical and coding problems
> - Improved intuition and creativity
> - Fewer emojis 🙃
This sounds like a significant upgrade to GPT-4o, albeit one where the release notes are limited to a single tweet.
ChatGPT-4o-latest (2025-0-26) just hit second place on [the LM Arena leaderboard](https://lmarena.ai/?leaderboard), behind only Gemini 2.5, so this really is an update worth knowing about.
The @OpenAIDevelopers account [confirmed](https://twitter.com/OpenAIDevs/status/1905335104211185999) that this is also now available in their API:
> `chatgpt-4o-latest` is now updated in the API, but stay tuned—we plan to bring these improvements to a dated model in the API in the coming weeks.
I [wrote about chatgpt-4o-latest](https://simonwillison.net/2025/Feb/17/llm/#chatgpt-4o-latest) last month - it's a model alias in the OpenAI API which provides access to the model used for ChatGPT, available since August 2024. It's priced at $5/million input and $15/million output - a step up from regular GPT-4o's $2.50/$10.
I'm glad they're going to make these changes available as a dated model release - the `chatgpt-4o-latest` alias is risky to build software against due to its tendency to change without warning.
A more appropriate place for this announcement would be the [OpenAI Platform Changelog](https://platform.openai.com/docs/changelog), but that's not had an update since the release of their new audio models on March 20th. |
2025-03-27 21:32:40+00:00 |
Thoughts on setting policy for new AI capabilities |
https://reservoirsamples.substack.com/p/thoughts-on-setting-policy-for-new |
Joanne Jang leads model behavior at OpenAI. Their release of GPT-4o image generation included some notable relaxation of OpenAI's policies concerning acceptable usage - I [noted some of those](https://simonwillison.net/2025/Mar/25/introducing-4o-image-generation/) the other day.
Joanne summarizes these changes like so:
> tl;dr we’re shifting from blanket refusals in sensitive areas to a more precise approach focused on preventing real-world harm. The goal is to embrace humility: recognizing how much we don't know, and positioning ourselves to adapt as we learn.
This point in particular resonated with me:
> - **Trusting user creativity over our own assumptions**. AI lab employees should not be the arbiters of what people should and shouldn’t be allowed to create.
A couple of years ago when OpenAI were the only AI lab with models that were worth spending time with it really did feel that San Francisco cultural values (which I relate to myself) were being pushed on the entire world. That cultural hegemony has been broken now by the increasing pool of global organizations that can produce models, but it's still reassuring to see the leading AI lab relaxing its approach here. |
2025-03-27 21:22:29+00:00 |
Nomic Embed Code: A State-of-the-Art Code Retriever |
https://www.nomic.ai/blog/posts/introducing-state-of-the-art-nomic-embed-code |
Nomic have released a new embedding model that specializes in code, based on their CoRNStack "large-scale high-quality training dataset specifically curated for code retrieval".
The [nomic-embed-code](https://huggingface.co/nomic-ai/nomic-embed-code) model is pretty large - 26.35GB - but the announcement also mentioned a much smaller model (released 5 months ago) called [CodeRankEmbed](https://huggingface.co/nomic-ai/CodeRankEmbed) which is just 521.60MB.
I missed that when it first came out, so I decided to give it a try using my [llm-sentence-transformers](https://github.com/simonw/llm-sentence-transformers) plugin for [LLM](https://llm.datasette.io/).
llm install llm-sentence-transformers
llm sentence-transformers register nomic-ai/CodeRankEmbed --trust-remote-code
Now I can run the model like this:
llm embed -m sentence-transformers/nomic-ai/CodeRankEmbed -c 'hello'
This outputs an array of 768 numbers, starting `[1.4794224500656128, -0.474479079246521, ...`.
Where this gets fun is combining it with my [Symbex tool](https://simonwillison.net/2023/Jun/18/symbex/) to create and then search embeddings for functions in a codebase.
I created an index for my LLM codebase like this:
cd llm
symbex '*' '*.*' --nl > code.txt
This creates a newline-separated JSON file of all of the functions (from `'*'`) and methods (from `'*.*'`) in the current directory - you can [see that here](https://gist.github.com/simonw/ac45c6638ea87942383e97c5cf69ae09).
Then I fed that into the [llm embed-multi](https://llm.datasette.io/en/stable/embeddings/cli.html#llm-embed-multi) command like this:
llm embed-multi \
-d code.db \
-m sentence-transformers/nomic-ai/CodeRankEmbed \
code code.txt \
--format nl \
--store \
--batch-size 10
I found the `--batch-size` was needed to prevent it from crashing with an error.
The above command creates a collection called `code` in a SQLite database called `code.db`.
Having run this command I can search for functions that match a specific search term in that `code` collection like this:
llm similar code -d code.db \
-c 'Represent this query for searching relevant code: install a plugin' | jq
That `"Represent this query for searching relevant code: "` prefix is required by the model. I pipe it through `jq` to make it a little more readable, which gives me [these results](https://gist.github.com/simonw/fdc1b48b20a99714200f5d3970b1dff4).
This `jq` recipe makes for a better output:
llm similar code -d code.db \
-c 'Represent this query for searching relevant code: install a plugin' | \
jq -r '.id + "\n\n" + .content + "\n--------\n"'
The output from that starts like so:
llm/cli.py:1776
@cli.command(name="plugins")
@click.option("--all", help="Include built-in default plugins", is_flag=True)
def plugins_list(all):
"List installed plugins"
click.echo(json.dumps(get_plugins(all), indent=2))
--------
llm/cli.py:1791
@cli.command()
@click.argument("packages", nargs=-1, required=False)
@click.option(
"-U", "--upgrade", is_flag=True, help="Upgrade packages to latest version"
)
...
def install(packages, upgrade, editable, force_reinstall, no_cache_dir):
"""Install packages from PyPI into the same environment as LLM"""
Getting this output was quite inconvenient, so I've [opened an issue](https://github.com/simonw/llm/issues/853). |
2025-03-27 20:03:56+00:00 |
Function calling with Gemma |
https://ai.google.dev/gemma/docs/capabilities/function-calling |
Google's Gemma 3 model (the 27B variant is particularly capable, I've been trying it out [via Ollama](https://ollama.com/library/gemma3)) supports function calling exclusively through prompt engineering. The official documentation describes two recommended prompts - both of them suggest that the tool definitions are passed in as JSON schema, but the way the model should request tool executions differs.
The first prompt uses Python-style function calling syntax:
> `You have access to functions. If you decide to invoke any of the function(s),
you MUST put it in the format of [func_name1(params_name1=params_value1, params_name2=params_value2...), func_name2(params)]`
>
> `You SHOULD NOT include any other text in the response if you call a function`
(Always love seeing CAPITALS for emphasis in prompts, makes me wonder if they proved to themselves that capitalization makes a difference in this case.)
The second variant uses JSON instead:
> `You have access to functions. If you decide to invoke any of the function(s),
you MUST put it in the format of {"name": function name, "parameters": dictionary of argument name and its value}`
>
> `You SHOULD NOT include any other text in the response if you call a function`
This is a neat illustration of the fact that all of these fancy tool using LLMs are still using effectively the same pattern as was described in [the ReAct paper](https://react-lm.github.io/) back in November 2022. Here's [my implementation of that pattern](https://til.simonwillison.net/llms/python-react-pattern) from March 2023. |
2025-03-26 20:23:06+00:00 |
Introducing 4o Image Generation |
https://openai.com/index/introducing-4o-image-generation/ |
When OpenAI first announced GPT-4o [back in May 2024](https://simonwillison.net/2024/May/13/gpt-4o/) one of the most exciting features was true multi-modality in that it could both input _and_ output audio and images. The "o" stood for "omni", and the image output examples [in that launch post](https://openai.com/index/hello-gpt-4o/) looked really impressive.
It's taken them over ten months (and Gemini [beat them to it](https://developers.googleblog.com/en/experiment-with-gemini-20-flash-native-image-generation/)) but today they're finally making those image generation abilities available, live right now in ChatGPT for paying customers.
My test prompt for any model that can manipulate incoming images is "Turn this into a selfie with a bear", because you should never take a selfie with a bear! I fed ChatGPT [this selfie](https://static.simonwillison.net/static/2025/selfie.jpg) and got back this result:
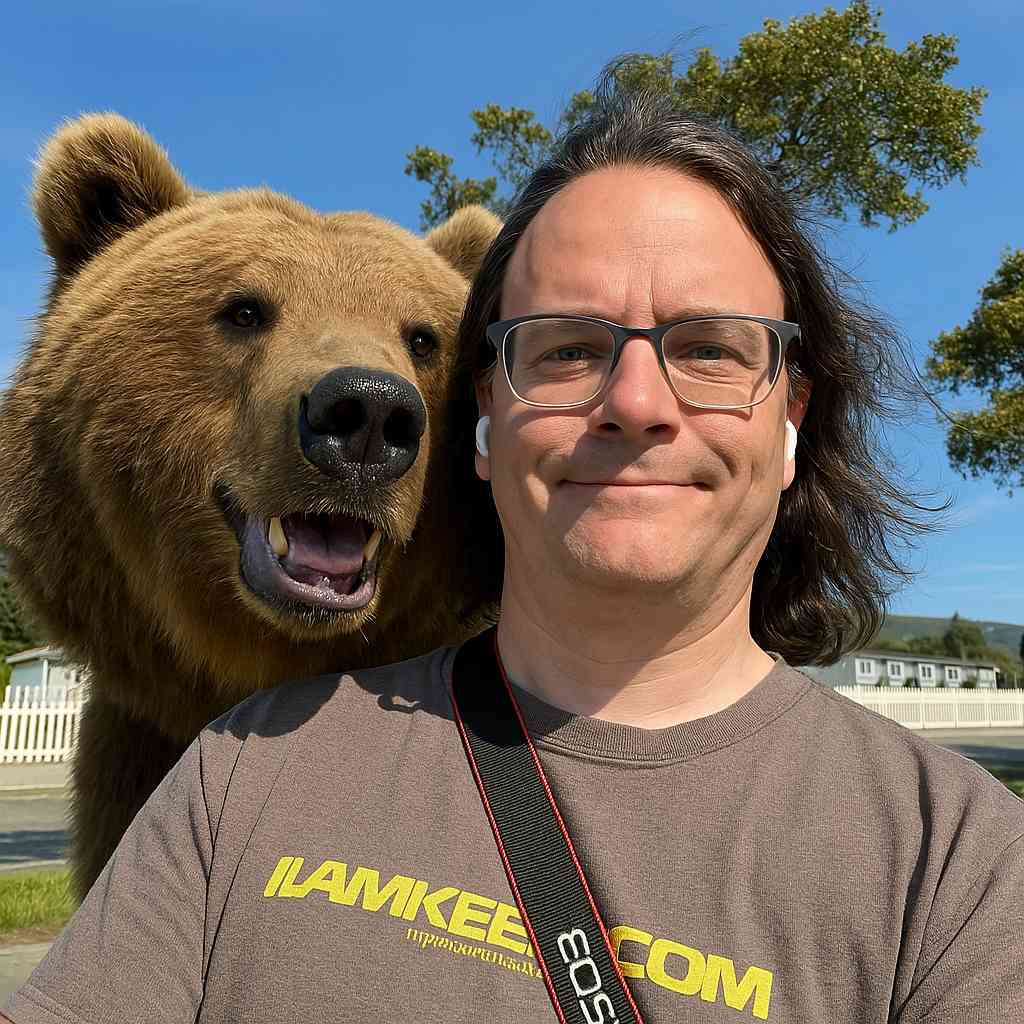
That's pretty great! It mangled the text on my T-Shirt (which says "LAWRENCE.COM" in a creative font) and added a second visible AirPod. It's very clearly me though, and that's definitely a bear.
There are plenty more examples in [OpenAI's launch post](https://openai.com/index/introducing-4o-image-generation/), but as usual the most interesting details are tucked away in [the updates to the system card](https://openai.com/index/gpt-4o-image-generation-system-card-addendum/). There's lots in there about their approach to safety and bias, including a section on "Ahistorical and Unrealistic Bias" which feels inspired by Gemini's [embarrassing early missteps](https://blog.google/products/gemini/gemini-image-generation-issue/).
One section that stood out to me is their approach to images of public figures. The new policy is much more permissive than for DALL-E - highlights mine:
> 4o image generation is capable, in many instances, of generating a depiction of a public figure based solely on a text prompt.
>
> **At launch, we are not blocking the capability to generate adult public figures** but are instead implementing the same safeguards that we have implemented for editing images of photorealistic uploads of people. For instance, this includes seeking to block the generation of photorealistic images of public figures who are minors and of material that violates our policies related to violence, hateful imagery, instructions for illicit activities, erotic content, and other areas. **Public figures who wish for their depiction not to be generated can opt out**.
>
> This approach is more fine-grained than the way we dealt with public figures in our DALL·E series of models, where we used technical mitigations intended to prevent any images of a public figure from being generated. **This change opens the possibility of helpful and beneficial uses in areas like educational, historical, satirical and political speech**. After launch, we will continue to monitor usage of this capability, evaluating our policies, and will adjust them if needed.
Given that "public figures who wish for their depiction not to be generated can opt out" I wonder if we'll see a stampede of public figures to do exactly that!
**Update**: There's significant confusion right now over this new feature because it is being rolled out gradually but older ChatGPT can still generate images using DALL-E instead... and there is no visual indication in the ChatGPT UI explaining which image generation method it used!
OpenAI made the same mistake last year [when they announced ChatGPT advanced voice mode](https://simonwillison.net/2024/May/15/chatgpt-in-4o-mode/) but failed to clarify that ChatGPT was still running the previous, less impressive voice implementation.
**Update 2**: Images created with DALL-E through the ChatGPT web interface now show a note with a warning:
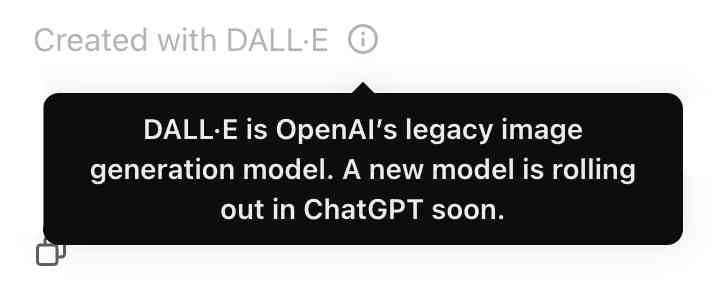 |
2025-03-25 21:11:23+00:00 |
shot-scraper 1.8 |
https://github.com/simonw/shot-scraper/releases/tag/1.8 |
I've added a new feature to [shot-scraper](https://shot-scraper.datasette.io/) that makes it easier to share scripts for other people to use with the [shot-scraper javascript]() command.
`shot-scraper javascript` lets you load up a web page in an invisible Chrome browser (via Playwright), execute some JavaScript against that page and output the results to your terminal. It's a fun way of running complex screen-scraping routines as part of a terminal session, or even chained together with other commands using pipes.
The `-i/--input` option lets you load that JavaScript from a file on disk - but now you can also use a `gh:` prefix to specify loading code from GitHub instead.
To quote [the release notes](https://github.com/simonw/shot-scraper/releases/tag/1.8):
> `shot-scraper javascript` can now optionally [load scripts hosted on GitHub](https://shot-scraper.datasette.io/en/stable/javascript.html#running-javascript-from-github) via the new `gh:` prefix to the `shot-scraper javascript -i/--input` option. [#173](https://github.com/simonw/shot-scraper/issues/173)
>
> Scripts can be referenced as `gh:username/repo/path/to/script.js` or, if the GitHub user has created a dedicated `shot-scraper-scripts` repository and placed scripts in the root of it, using `gh:username/name-of-script`.
>
> For example, to run this [readability.js](https://github.com/simonw/shot-scraper-scripts/blob/main/readability.js) script against any web page you can use the following:
>
> shot-scraper javascript --input gh:simonw/readability \
> https://simonwillison.net/2025/Mar/24/qwen25-vl-32b/
The [output from that example](https://gist.github.com/simonw/60e196ec39a5a75dcabfd75fbe911a4c) starts like this:
<div class="highlight highlight-source-json"><pre>{
<span class="pl-ent">"title"</span>: <span class="pl-s"><span class="pl-pds">"</span>Qwen2.5-VL-32B: Smarter and Lighter<span class="pl-pds">"</span></span>,
<span class="pl-ent">"byline"</span>: <span class="pl-s"><span class="pl-pds">"</span>Simon Willison<span class="pl-pds">"</span></span>,
<span class="pl-ent">"dir"</span>: <span class="pl-c1">null</span>,
<span class="pl-ent">"lang"</span>: <span class="pl-s"><span class="pl-pds">"</span>en-gb<span class="pl-pds">"</span></span>,
<span class="pl-ent">"content"</span>: <span class="pl-s"><span class="pl-pds">"</span><div id=<span class="pl-cce">\"</span>readability-page-1<span class="pl-cce">\"...</span></pre></div>
My [simonw/shot-scraper-scripts](https://github.com/simonw/shot-scraper-scripts) repo only has that one file in it so far, but I'm looking forward to growing that collection and hopefully seeing other people create and share their own `shot-scraper-scripts` repos as well.
This feature is an imitation of [a similar feature](https://github.com/simonw/llm/issues/809) that's coming in the next release of LLM. |
2025-03-25 01:59:38+00:00 |
microsoft/playwright-mcp |
https://github.com/microsoft/playwright-mcp |
The Playwright team at Microsoft have released an MCP ([Model Context Protocol](https://github.com/microsoft/playwright-mcp)) server wrapping Playwright, and it's pretty fascinating.
They implemented it on top of the Chrome accessibility tree, so MCP clients (such as the Claude Desktop app) can use it to drive an automated browser and use the accessibility tree to read and navigate pages that they visit.
Trying it out is quite easy if you have Claude Desktop and Node.js installed already. Edit your `claude_desktop_config.json` file:
code ~/Library/Application\ Support/Claude/claude_desktop_config.json
And add this:
<div class="highlight highlight-source-json"><pre>{
<span class="pl-ent">"mcpServers"</span>: {
<span class="pl-ent">"playwright"</span>: {
<span class="pl-ent">"command"</span>: <span class="pl-s"><span class="pl-pds">"</span>npx<span class="pl-pds">"</span></span>,
<span class="pl-ent">"args"</span>: [
<span class="pl-s"><span class="pl-pds">"</span>@playwright/mcp@latest<span class="pl-pds">"</span></span>
]
}
}
}</pre></div>
Now when you launch Claude Desktop various new browser automation tools will be available to it, and you can tell Claude to navigate to a website and interact with it.
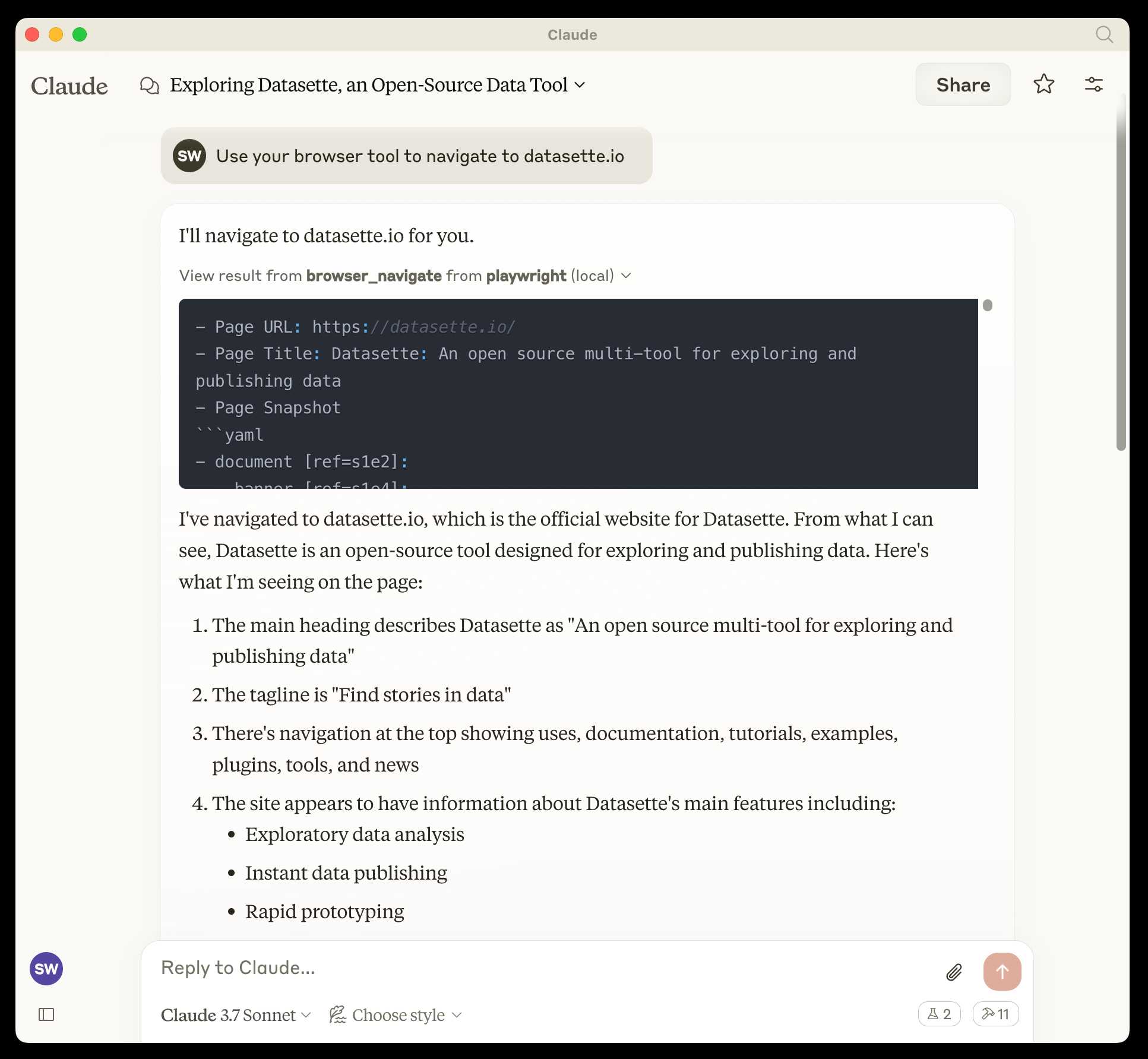
I ran the following to get a list of the available tools:
cd /tmp
git clone https://github.com/microsoft/playwright-mcp
cd playwright-mcp/src/tools
files-to-prompt . | llm -m claude-3.7-sonnet \
'Output a detailed description of these tools'
The [full output is here](https://gist.github.com/simonw/69200999149221c549c1f62e7befa20f), but here's the truncated tool list:
> #### Navigation Tools (`common.ts`)
>
> - **browser_navigate**: Navigate to a specific URL
> - **browser_go_back**: Navigate back in browser history
> - **browser_go_forward**: Navigate forward in browser history
> - **browser_wait**: Wait for a specified time in seconds
> - **browser_press_key**: Press a keyboard key
> - **browser_save_as_pdf**: Save current page as PDF
> - **browser_close**: Close the current page
>
>
> #### Screenshot and Mouse Tools (`screenshot.ts`)
>
> - **browser_screenshot**: Take a screenshot of the current page
> - **browser_move_mouse**: Move mouse to specific coordinates
> - **browser_click** (coordinate-based): Click at specific x,y coordinates
> - **browser_drag** (coordinate-based): Drag mouse from one position to another
> - **browser_type** (keyboard): Type text and optionally submit
>
>
> #### Accessibility Snapshot Tools (`snapshot.ts`)
>
> - **browser_snapshot**: Capture accessibility structure of the page
> - **browser_click** (element-based): Click on a specific element using accessibility reference
> - **browser_drag** (element-based): Drag between two elements
> - **browser_hover**: Hover over an element
> - **browser_type** (element-based): Type text into a specific element |
2025-03-25 01:40:05+00:00 |
Qwen2.5-VL-32B: Smarter and Lighter |
https://qwenlm.github.io/blog/qwen2.5-vl-32b/ |
The second big open weight LLM release from China today - the first being [DeepSeek v3-0324](https://simonwillison.net/2025/Mar/24/deepseek/).
Qwen's previous vision model was Qwen2.5 VL, [released in January](https://simonwillison.net/2025/Jan/27/qwen25-vl-qwen25-vl-qwen25-vl/) in 3B, 7B and 72B sizes.
Today's Apache 2.0 licensed release is a 32B model, which is quickly becoming my personal favourite model size - large enough to have GPT-4-class capabilities, but small enough that on my 64GB Mac there's still enough RAM for me to run other memory-hungry applications like Firefox and VS Code.
Qwen claim that the new model (when compared to their previous 2.5 VL family) can "align more closely with human preferences", is better at "mathematical reasoning" and provides "enhanced accuracy and detailed analysis in tasks such as image parsing, content recognition, and visual logic deduction".
They also offer some presumably carefully selected benchmark results showing it out-performing Gemma 3-27B, Mistral Small 3.1 24B and GPT-4o-0513 (there have been two more recent GPT-4o releases since that one, 2024-08-16 and 2024-11-20).
As usual, Prince Canuma had MLX versions of the models live within hours of the release, in [4 bit](https://huggingface.co/mlx-community/Qwen2.5-VL-32B-Instruct-4bit), [6 bit](https://huggingface.co/mlx-community/Qwen2.5-VL-32B-Instruct-6bit), [8 bit](https://huggingface.co/mlx-community/Qwen2.5-VL-32B-Instruct-8bit), and [bf16](https://huggingface.co/mlx-community/Qwen2.5-VL-32B-Instruct-bf16) variants.
I ran the 4bit version (a 18GB model download) using `uv` and Prince's [mlx-vlm](https://github.com/Blaizzy/mlx-vlm) like this:
<div class="highlight highlight-source-shell"><pre>uv run --with <span class="pl-s"><span class="pl-pds">'</span>numpy<2<span class="pl-pds">'</span></span> --with mlx-vlm \
python -m mlx_vlm.generate \
--model mlx-community/Qwen2.5-VL-32B-Instruct-4bit \
--max-tokens 1000 \
--temperature 0.0 \
--prompt <span class="pl-s"><span class="pl-pds">"</span>Describe this image.<span class="pl-pds">"</span></span> \
--image Mpaboundrycdfw-1.png</pre></div>
Here's the image:
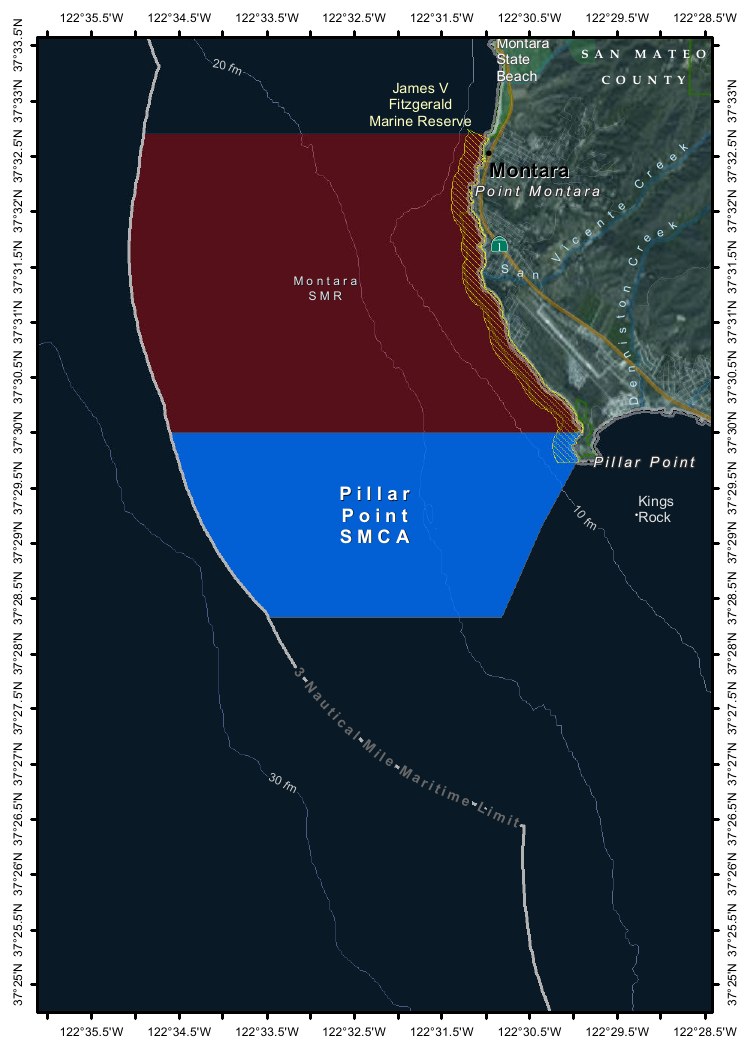
And here's the resulting description, which I found to be _very_ impressive:
<blockquote>
<p>This image appears to be a map highlighting specific areas along the coastline, likely in California, based on the geographical features and place names. Here is a detailed description of the image:</p>
<ol>
<li>
<p><strong>Geographic Features</strong>:</p>
<ul>
<li>The map shows a section of the coastline, with land on the right and the sea on the left.</li>
<li>The land area includes parts of SanMateo County, as noted in the top-right corner.</li>
<li>The coastline features several named locations, including:
<ul>
<li><strong>Point Montara</strong></li>
<li><strong>Pillar Point</strong></li>
<li><strong>Kings Rock</strong></li>
<li><strong>VicenteCreek</strong></li>
<li><strong>DennistonCreek</strong></li>
<li><strong>State Beach</strong></li>
</ul>
</li>
</ul>
</li>
<li>
<p><strong>Highlighted Areas</strong>:</p>
<ul>
<li>The map contains two distinct areas marked with different colors:
<ul>
<li>
<strong>Red Area (Top Section)</strong>
<ul>
<li>Labeled as "MONTARA" and "MONTARA M R" (possibly referring to a "Marine Reserves" or similar protected area).</li>
<li>This area is marked with a dark red color and extends from the coastline inland.</li>
</ul>
</li>
<li>
<strong>Blue Area (Bottom Section)</strong>
<ul>
<li>Labeled as "PILLAR" and "PILLAR P O I N T" along with "SMCA" (Special Management Area).</li>
<li>This area is marked with a blue color and is located closer to the coastline, including the area around "Pillar Point."</li>
</ul>
</li>
</ul>
</li>
</ul>
</li>
<li>
<p><strong>Depth Contours</strong>:</p>
<ul>
<li>The map includes depth markings in the sea, indicating the bathymetrical features:
<ul>
<li>
<strong>20 fathom (fm) line</strong> is marked in the upper-left part of the sea.</li>
<li>
<strong>10 fathom (fm) line</strong> is marked closer to the coastline.</li>
<li>
<strong>30 fathom (fm) line</strong> is marked further out to sea.</li>
</ul>
</li>
</ul>
</li>
<li>
<p><strong>Other Features</strong>:</p>
<ul>
<li>
<strong>State Beach</strong>: Marked near the top-right, indicating a protected recreational area.</li>
<li>
<strong>Kings Rock</strong>: A prominent feature near the coastline, likely a rocky outcropping.</li>
<li>
<strong>Creeks</strong>: The map shows several creeks, including <strong>VicenteCreek</strong> and <strong>DennistonCreek</strong>, which flow into the sea.</li>
</ul>
</li>
<li>
<p><strong>Protected Areas</strong>:</p>
<ul>
<li>The map highlights specific protected areas:
<ul>
<li>
<strong>Marine Reserves</strong>:
<ul>
<li>"MONTARA M R" (Marine Reserves) in red.</li>
</ul>
</li>
<li>
<strong>Special Management Area (SMCA)</strong>
<ul>
<li>"PILLAR P O I N T" in blue, indicating a Special Management Area.</li>
</ul>
</li>
</ul>
</li>
</ul>
</li>
<li>
<p><strong>Grid and Coordinates</strong>:</p>
<ul>
<li>The map includes a grid with latitude and longitude markings:
<ul>
<li>Latitude ranges from approximately 37°25'N to 37°35'N.</li>
<li>Longitude ranges from approximately 122°22.5'W to 122°35.5'W.</li>
</ul>
</li>
</ul>
</li>
<li>
<p><strong>Topography</strong>:</p>
<ul>
<li>The land area shows topographic features, including elevations and vegetation, with green areas indicating higher elevations or vegetated land.</li>
</ul>
</li>
<li>
<p><strong>Other Labels</strong>:</p>
<ul>
<li>
<strong>"SMR"</strong>: Likely stands for "State Managed Reserves."</li>
<li>
<strong>"SMCA"</strong>: Likely stands for "Special Management Control Area."</li>
</ul>
</li>
</ol>
<p>In summary, this map highlights specific protected areas along the coastline, including a red "Marine Reserves" area and a blue "Special Management Area" near "Pillar Point." The map also includes depth markings, geographical features, and place names, providing a detailed view of the region's natural and protected areas.</p>
</blockquote>
It included the following runtime statistics:
Prompt: 1051 tokens, 111.985 tokens-per-sec
Generation: 760 tokens, 17.328 tokens-per-sec
Peak memory: 21.110 GB |
2025-03-24 22:43:22+00:00 |